Processing free text for a better risk picture in accelerated decisions

properties.trackTitle
properties.trackSubtitle
One challenge in accelerated life underwriting is getting a complete picture of an applicant’s health, quickly and non-invasively. Capturing applicant information with free-text boxes at the point of sale adds additional context, but digesting this data is often a manual process that slows down turnaround times. To tackle this issue, the team at alitheia, Munich Re’s risk assessment and instant decisioning system, created a process to map select free-text comments and assign a medical severity that feeds into alitheia’s rules engine for decisioning. Already in production, this solution has increased straight-through processing (STP) rates by approximately 5%.
In this Q&A, Adnan Haque, 2nd Vice President Integrated Analytics, Melissa Butcher, Senior Underwriting Risk Specialist, and Magd Bayoumi, Senior Machine Learning Engineer, discuss how the interdisciplinary team at alitheia worked together to create this solution.
Q: It’s great to get both the underwriting and technical perspective on improving straight-through-processing rates in life underwriting. Can you describe the problem you are solving from your point of view?
Melissa Butcher: Life insurance companies continually enhance their digital processes to improve the customer experience by reducing the time it takes for a decision. The quicker the decision, the higher the close rate. However, according to Munich Re’s latest accelerated underwriting survey of U.S. life carriers, only 10-15% of cases were automatically decisioned at the point of sale.
One way to increase automated decision rates is to ask fewer questions, and many accelerated programs use this simplified approach, charging slightly higher prices to compensate for the unknown risks. In alitheia, our goal is to capture an accurate picture of applicant risk. Instead of asking fewer questions, this project focused on improving application processing, starting with “catch-all” questions and their associated free-text responses.
Typical life insurance applications include text boxes that allow applicants to provide additional details on their health status. This information provides essential context to underwriters, confirming or contradicting structured answers or adding details describing a condition's severity. However, applicants often enter a procedure or condition that is not medically severe. For example, it’s common for individuals to disclose benign issues such as seasonal allergies or pregnancy hospitalizations. We wanted to find a way to digitally process this information so that only the severe cases are sent for underwriting review and the medically insignificant cases are passed through.
Adnan Haque: Our goal was to use natural language processing and machine learning to identify keywords in the free-form text. While this capability is not new, in life insurance underwriting it poses a tricky problem. Not only must the free-form data be read and classified, but it must also be assigned a medical severity.
To do this, we needed to solve three significant challenges: First, because of its nature as unstructured information, free text is challenging to read and automate. The possible responses are unlimited. Second, the text can be inconsistent since an applicant could easily misspell a word or use abbreviations for impairments. Finally, the data must be categorized according to medical risk. If the system can’t tell if the answer is medically significant, the application gets kicked out of the accelerated workflow and sent to an underwriter for manual review.
Q: So, how did you approach the project?
Melissa Butcher: We developed a methodology for the type of keywords we wanted to capture and assess. No matter the condition, underwriters generally ask the same questions: “What do you have?” “When was it diagnosed?” and “How is it treated?” With that in mind, we focused on three areas to map – diagnosis codes, prescriptions, and procedure codes – since together they provide an indication of medical severity.
Our underwriters got to work classifying these codes and medications, which was no easy task. To date, they have mapped over 80,000 keywords to mark as ‘safe’ or ‘potentially unsafe.’ It’s a delicate balance – you don’t want people to fall out of the automated process for minor conditions, but it is essential to capture any major conditions properly.
Magd Bayoumi: Munich Re has been building models to process free text in attending physician statements for over five years. We wanted to apply that knowledge to extract and codify the relevant keywords to answer the question: “Is it significant?”
Our team created custom natural language understanding models to extract and process free text based on established rules. Once an application is complete in the alitheia risk assessment and decisioning system, any free-form text is processed to identify the medical text and assess its risk. Machine learning technologies, including word embedding, named entity recognition, and code resolution models are applied to grasp the context and identify the medical keywords in the free text. The keywords are compared to the diagnosis, prescription, and procedure codes database to assign a risk assessment of safe or potentially unsafe. This risk assessment can then be fed into alitheia’s medical classification framework, triggering downstream rules and models.
Here are some examples. An applicant might enter, ‘I have a bee sting allergy and take ephedrine.’ These two phrases, ‘bee sting allergy’ and ‘ephedrine,’ are identified as keywords of potential medical importance. This response would be marked as safe, and decisioning could proceed uninterrupted. Another person might enter, ‘Lisinopril for hypertension and metformin for diabetes.’ Our model would identify two medications and two conditions. These would be marked as potentially unsafe and go into an underwriting queue for human assessment.
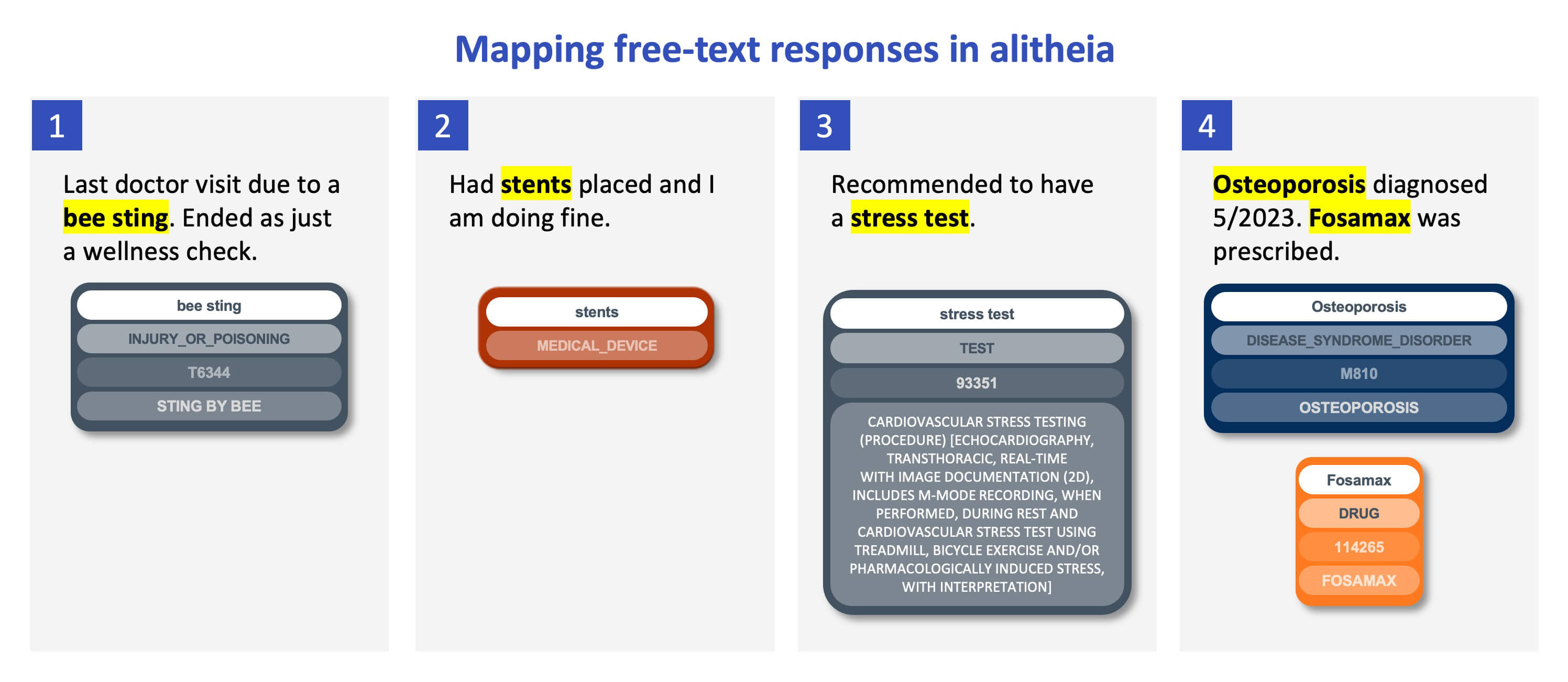
Q: This solution is live on alitheia. What has been the impact?
Melissa Butcher: The results have exceeded expectations. So far, nearly 50% of the free text answers eligible for keyword mapping were flagged as safe without a material impact on the decision. STP rates were improved by an average of 17% on eligible cases. This means that our clients can now instant-decision more cases than previously possible, leading to a better client experience and higher close rates.
In addition, our clients also appreciate no longer having to wait for application re-filing to update application questions in an attempt to get more details since we can allow for free-form text responses in alitheia. This could also help us restructure filed questions to avoid any behavioral biases and allow for more free-text responses.
Magd Bayoumi: We are very happy with how the models are performing. Our extensive testing and close monitoring of current cases indicate that tagging medical terms and assigning codes exceeds our initial internal expectations. Our mortality is unaffected, and STP increased as well.
Q: This is a significant advancement for accelerated underwriting. What’s the next evolution?
Magd Bayoumi: We’re working on further improvements to refine our data mapping models and apply them to assign severity for additional inputs in an online application, such as “last doctor’s visit.” Another enhancement is connecting application data to other information already in alitheia, which should improve user experience by making the application process more efficient.
This technology also provides the framework for structuring medical text in electronic health records (EHRs). We’re already leveraging it within EHRs on a post-issue basis and are rolling out this technology on clinical notes for EHRs. Making EHRs readable in real-time is a very exciting advancement in accelerated underwriting.
Adnan Haque: As Magd mentioned, we’re making additional refinements to help us get to the actual risk, allowing our clients to increase their offer rates. Currently, the instant life insurance offer rate with no human involvement is 10% to 15%. If a human intervenes, it is 40%-50%, according to Munich Re’s internal research. With alitheia, we aim to get to 50%+ and replace an invasive process without making it riskier. This ongoing team effort relies on underwriting and technical expertise to improve accelerated underwriting for our clients and the industry.
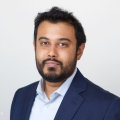
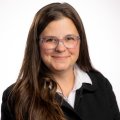
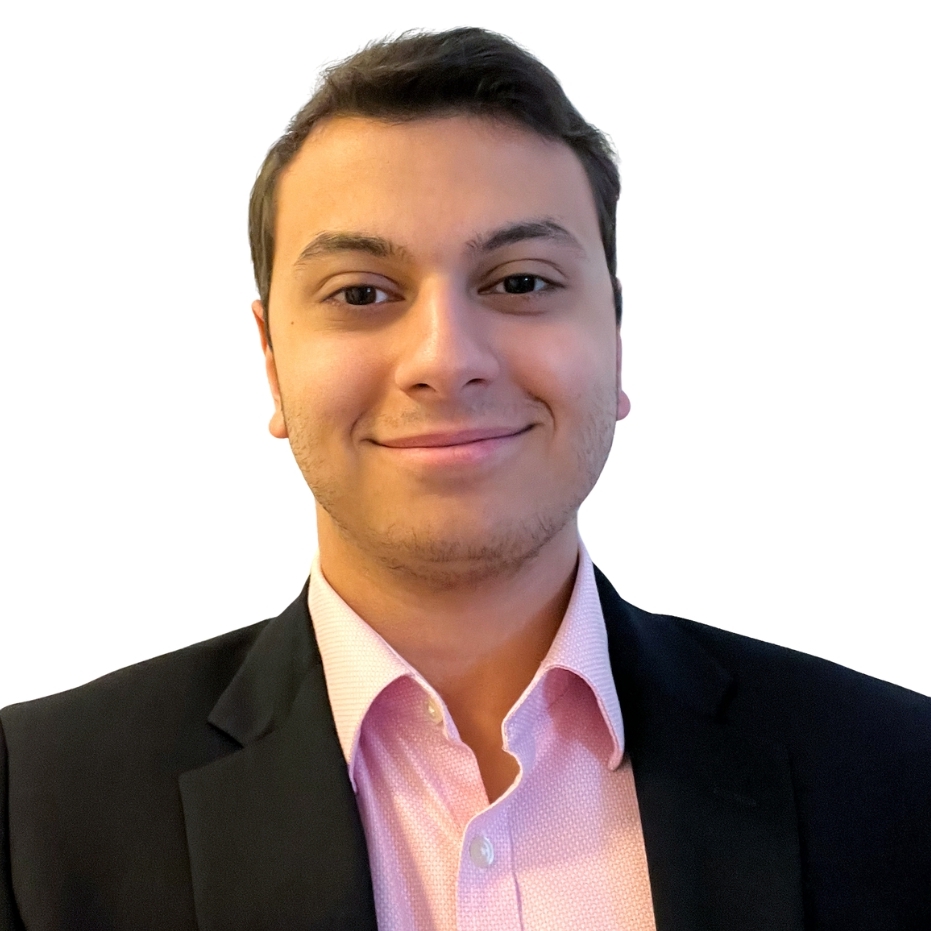
This may interest you
Newsletter
properties.trackTitle
properties.trackSubtitle