
properties.trackTitle
properties.trackSubtitle
Using third-party longitudinal data in life insurance underwriting
The growing prevalence of accelerated underwriting (AUW) programs in the life insurance industry in the last decade has led carriers to explore the use of third-party data sources as a substitute for traditional requirements such as blood, urine, paramedical exams, and attending physician statements (APS). In the U.S., a range of well-established third-party data sources are available, including prescription and medical claims history databases, aggregated lab medical test results, and motor vehicle records.
The simultaneous evolution of wearable technology offers a significant opportunity to explore and analyze previously underutilized variables in the underwriting process. Wearables capture a variety of data on health, habits, and lifestyle, such as physical activity, sleep duration, and heart rate. Beyond AUW, wearable data can be applied in traditional full underwriting (FUW) and customer wellness initiatives due to the immediate feedback it provides.
The UK Biobank, a large database including several health-related variables, is a frequently cited data source in recent research on mortality and morbidity.1 Munich Re Life US collaborated with Klarity, a UK-based health data analytics firm, on a study of the UK Biobank data to assess the potential advantages of adopting third-party longitudinal data, including from wearables, in underwriting.2
Our research is presented in a series of white papers that analyze the mortality impact of different variables in the UK Biobank, including:
Background and methodology: Applying the UK Biobank data to life insurance underwriting
UK Biobank
The UK Biobank is a prospective longitudinal study of de-identified individuals drawn from the UK general population who volunteered to have their data collected across a broad range of core categories.3 The database was created to aid research initiatives supporting the diagnosis, prevention, and treatment of disease. Researchers are required to obtain permission to secure access to this data.
Figure 1 shows the major UK Biobank categories from which Klarity extracted data for this research. Other categories of data in the UK Biobank, including cognitive function, imaging measures, geographical measures, and genotype results, are not part of this study.

Klarity extracted and shared the de-identified data set with Munich Re Life US after creating new variables summarizing participant medical information. The data set included 502,369 individuals whose study entry dates range from 2006 to 2010 and were followed through the end of 2022, including 37,897 deaths. Participants were followed for an average period of 13.5 years.
Table 1 shows the salient (not exhaustive) predictor variables from this dataset included in our analysis. Our focus was to study the impact of these variables on mortality and apply the insights to refine life insurance underwriting.

Assumptions to simulate an insurable pool
Munich Re applied screening criteria to the initial dataset of UK Biobank participants to create a subset of healthier lives that mirrored a typical insurable pool.
The “insurable” block excluded participants with:
- BMI, blood pressure, total cholesterol, and gamma-glutamyl transferase (GGT) values above insurable limits.
- Known medical history of cardiovascular disease (including stroke), cancer, lung disease, dementia, hepatitis B, hepatitis C, HIV and other rare terminal diseases.
- Index of multiple deprivation (IMD) in the worst 15%. This measure is a broad indicator of socio-economic status covering seven categories at the post-code level – crime, income, education, employment, health deprivation, living environment, and barrier to housing and services.
Table 2 summarizes attributes of the overall “population” and the subset of “insurable” data. “Smoker %” refers to the percentage of “current” smokers. “Never” and “former” smokers together comprise nonsmokers.

Considerations for interpreting the study results
Features unique to this “insurable” pool Include:
- Few individuals were under the age of 40 at the time of study entry.
- Study participants were not underwritten for life insurance so anti-selection is not a factor.
- The screening criteria applied to generate the “insurable” pool were based on lab tests, physical exams, deprivation measures, and ICD-10 (diagnosis) codes from the hospital summaries.
- Multiple parameters of interest, including step count and physical measurements, were measured in a well-calibrated environment which may not always be possible to replicate in life insurance underwriting. Physical activity readings were taken from participants who wore wrist accelerometers for at least seven days. Grip strength readings were obtained from hand dynamometers.
- Analysis of the physical activity data (in the next paper on wearable physical activity data) revealed that individuals consenting to provide activity data have better mortality than those without measurements. This suggests that there is a certain amount of self-selection, where those who consented to provide measurable activity data were already more likely to be physically active. An insurance program in which everyone consents to provide activity data may have a lower proportion of members with high levels of physical activity.
About Klarity
References
Contact

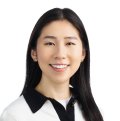
Read the rest of the series
Newsletter
properties.trackTitle
properties.trackSubtitle